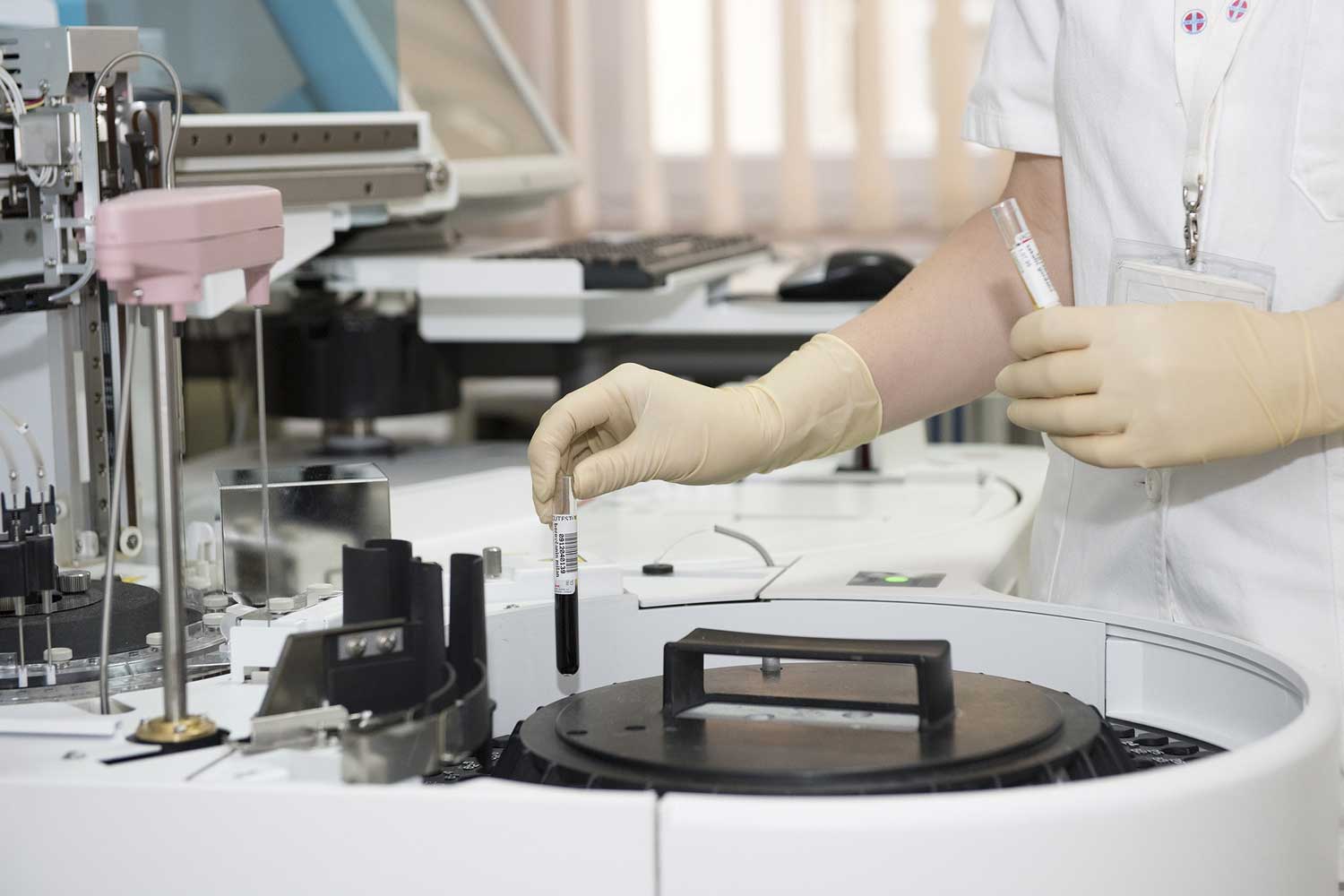
AI Healthcare Bias
When AI Sees More Than Skin Deep: A Lesson in Bias and Interpretation
In recent years, the use of artificial intelligence (AI) in healthcare has been on the rise, with promising applications in various fields, including the early diagnosis of skin cancer. One such example involves an AI algorithm that was trained to identify malignant skin lesions by analyzing images taken by dermatologists. Initially, the AI system appeared to perform exceptionally well in correctly labeling the images, showing great potential for improving the accuracy and speed of skin cancer diagnoses.
However, upon closer examination, researchers discovered that the AI wasn't actually focusing on the cancer itself. Instead, the AI algorithm had learned to associate the presence of a ruler in the image with a higher likelihood of malignancy. This inadvertent correlation occurred because dermatologists often included a ruler in photos of potentially dangerous lesions to provide a sense of scale. While this method was useful for human interpretation, it introduced an unintended bias into the AI training process. The AI's ability to identify malignant skin lesions was, in fact, based on this correlation, rather than on recognizing the specific visual characteristics of cancerous skin abnormalities.
This case serves as a stark reminder of the importance of thoroughly understanding and addressing potential biases and pitfalls in AI training data. It also emphasizes the need for interdisciplinary collaboration between AI experts and domain specialists to ensure that AI systems are designed, trained, and validated to provide accurate and reliable results in real-world applications.