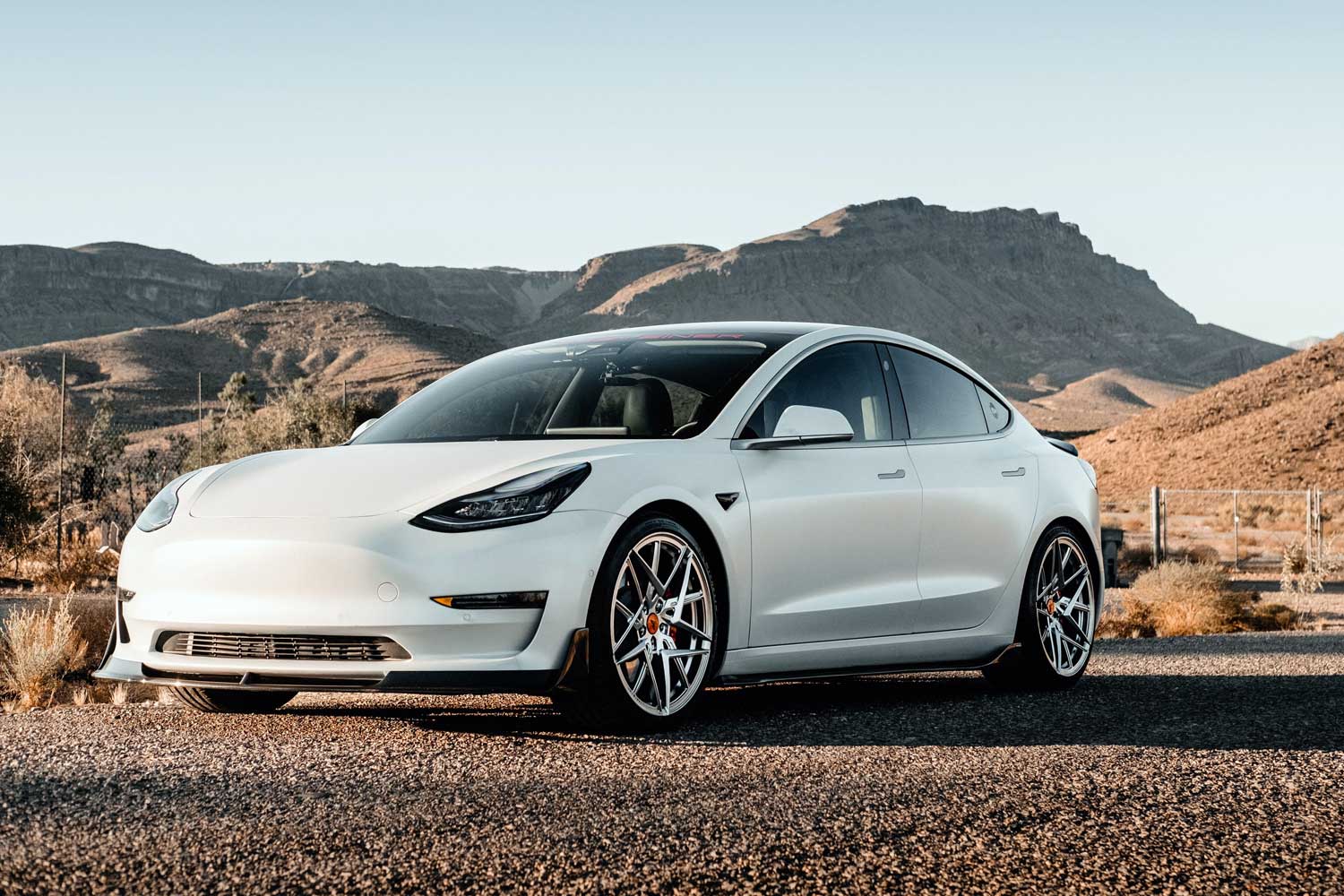
How Tesla and Tweet data could help Elon Musk achieve AGI
With his dual-data strategy, Musk isn't merely a participant in the AGI race; he's poised to lead it.
Musk's Unique Advantage in the Race to AGI
The pursuit of Artificial General Intelligence (AGI) – machines that can mirror human-like physical and intellectual capabilities – is advancing rapidly. Central to this evolution is the indispensable component: data.
While tech giants such as Google and Microsoft have made impressive strides in AI, drawing from vast data sources such as their their search engines, email and cloud services, their primary data has been chatbot-centric.
In contrast, Elon Musk possesses two potent real-time data streams: images from Tesla's self-driving cars and billions of posts on Twitter each week. This positions him distinctively for AGI, potentially ahead of Google and Microsoft.
Twitter: Tapping into Humanity's Digital Pulse
Musk's acquisition of Twitter goes beyond social media; it's about accessing a real-time reflection of humanity. With trillions of tweets already existing and an additional 500 million being added daily, Twitter provides a window into global conversations, trends, sentiments, and linguistic nuances. This vast dataset serves as an invaluable training ground for chatbots, refining their interactions based on immediate human feedback. Interestingly, the potential of this data wasn't Musk's primary motivation behind the Twitter acquisition; it was a serendipitous discovery he made post-purchase.
Recognising the goldmine he had inadvertently accessed, Musk quickly strategised to capitalise on this asset. He explored avenues to monetise this data stream, not just for revenue but also as a strategic move to potentially challenge AI heavyweights like Google and Microsoft.
Tesla: Navigating the Real World through AI's Eyes
Beyond the digital realm, Tesla adds another layer to Musk's AGI vision. Each day, Tesla processes an astounding 160 billion video frames from its fleet. This data captures the intricacies of human navigation in real-world scenarios, offering invaluable insights for training AI in physical tasks. While text data from Twitter informs chatbots, Tesla's video data bridges the gap between virtual assistants and tangible robots.
Beyond Electric Vehicles: Tesla's Grand AI Vision
Elon Musk's aspirations for Tesla go beyond electric vehicles. His ventures into various AI domains, from self-driving cars to the humanoid robot Optimus and the innovative Neuralink brain-machine interface, have been groundbreaking. His overarching ambition is to develop a self-driving car system that learns from human behaviour, rather than just adhering to preset rules.
For years, Tesla's autopilot operated on a rules-based approach. It processed visual data from the car's cameras, identifying elements like lane markings, pedestrians, vehicles, and traffic signals captured by its 8 cameras. The software then implemented a set of predefined rules such as stop if the traffic light is red, go when it is green, keep inside the the lane markers, dont cross double lines into oncoming traffic, only drive through an intersection when no cars are coming fast enough to hit you etc. To handle these intricate scenarios, Tesla's engineers meticulously crafted and updated hundreds of thousands of lines of C+ code to apply these rules to the situations the cars could find themselves in.
The Neural Network Path Planner project introduced a transformative layer. Instead of determining the car's path solely based on rules, it also considers a neural network that learns from millions of human examples. Musk recognised the risk that using all the available images could mean the system would only be as good as the average driver, so he instructed the human labellers, many based in Buffalo, NY to review and select only the images displaying the actions that a 5* Uber driver would take. Tesla has replaced the human labelling process with auto-labeling training software to dramatically accelerate this process. By early 2023, the Neural Network Planner had analysed an impressive 10 million video frames from Tesla vehicles.
Metrics to keep the team on target were crucial. Musk selected the metric tracking the miles Tesla vehicles could travel without human intervention. Giant screens were placed by the engineers' desks to display this, and any increase in interventions (such as drivers grabbing the wheel during a lane change or a turn into a complex intersection) led to the engineers working with both the rules and the Neural Network Planner to make a fix. Every time they fixed a problem, the engineers got to bang a gong, adding a touch of gamification to the process.
Tesla's advantage became evident when the neural network began working well after training on 1 million video clips and then excelling after training on 1.5 million video clips. With a fleet of over 2 million Teslas around the world, collecting billions of video frames daily, Tesla's image data collection capability is unparalleled.
Conclusion: Musk's Dual-Data Strategy – A Game Changer in AGI
The race to AGI is about more than mimicking human conversation or movement; it's about replicating the entirety of human experience. With his dual-data strategy, Musk isn't merely a participant in the AGI race; he's poised to lead it. As Tesla continues to harness its unmatched data capabilities and Twitter captures the essence of human conversation, the convergence of these data streams under Musk's visionary leadership promises a transformative future for AGI.