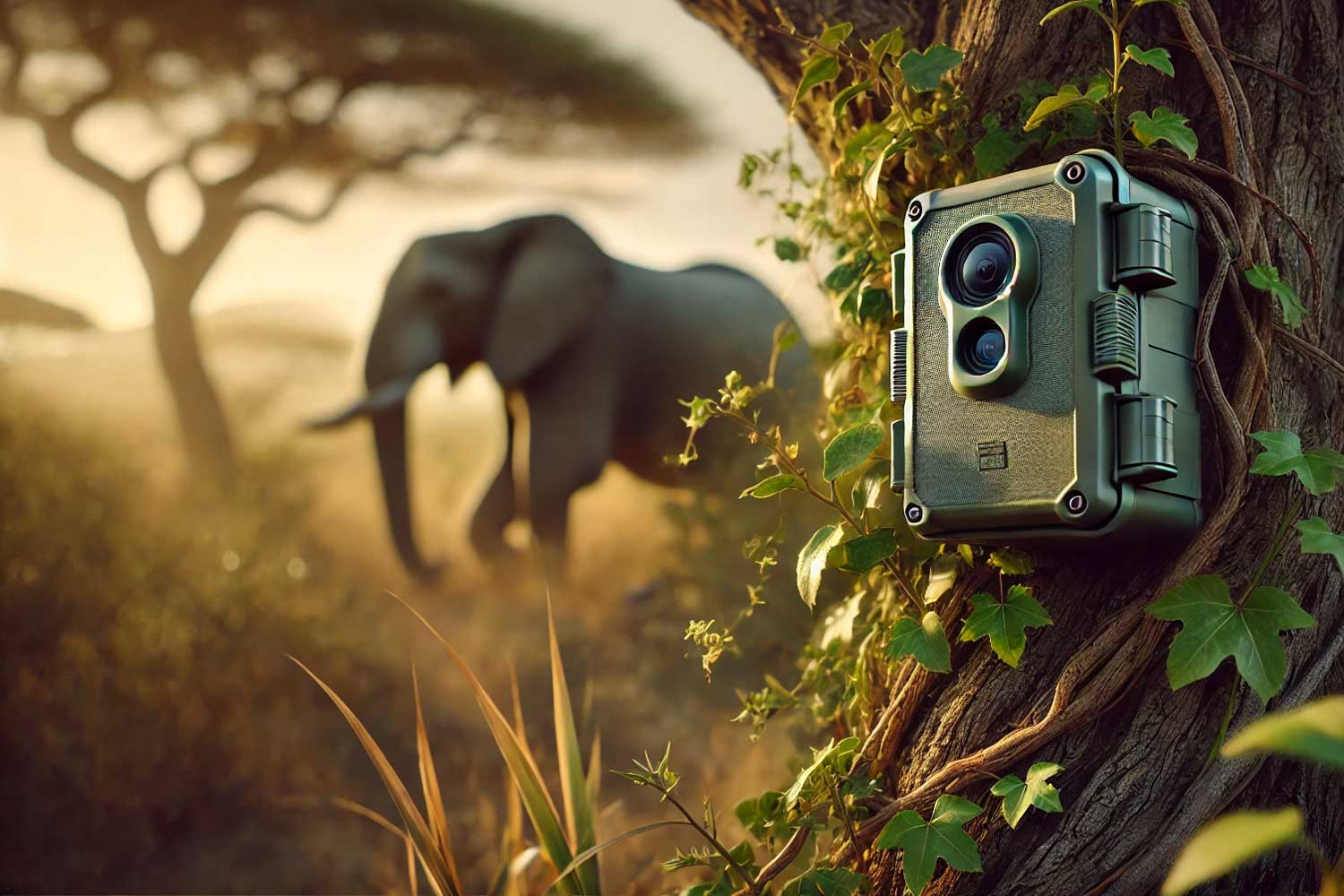
Tackling Wildlife Crime with AI and Satellite Connectivity
Instant Detect 2.0 is a ZSL-led project combining AI camera traps with satellite connectivity. Designed for remote areas, it filters threat images in real time and alerts rangers, helping to combat wildlife crime and protect endangered species worldwide.
Detecting Poachers from Space: How Instant Detect 2.0 is Transforming Conservation with AI
The fight against wildlife crime has taken to the skies, the seas and now—crucially—to space. While many conservation tools rely on drones and traditional camera traps, the Zoological Society of London (ZSL) has been quietly pioneering a new system called Instant Detect 2.0. This technology aims to deliver real-time intelligence to rangers in some of the world’s most remote locations, relying on satellite transmissions and onboard artificial intelligence to spot potential threats before it’s too late.
Confronting the Challenge of Remote Conservation
Wildlife rangers often face daunting challenges in their efforts to protect endangered species. Poachers are increasingly sophisticated, exploiting regions that are difficult to patrol and even harder to monitor using conventional surveillance tools. In the heat of the African savannah or the desolate expanses of Antarctic wilderness, mobile phone signals are non-existent and harsh climates can degrade standard camera equipment.
Instant Detect 2.0 was conceived to overcome precisely these hurdles. By harnessing satellite connectivity, the system ensures that images and alerts can be transmitted in near-real-time from anywhere on the planet. This means rangers no longer have to rely on patchy cell coverage or physically retrieving camera trap memory cards (which can entail days of travel). Instead, they receive instant notifications and can respond swiftly to suspicious activity.
Inside Instant Detect 2.0: Hardware Meets AI
At its core, Instant Detect 2.0 comprises robust camera traps equipped with motion sensors, powered by low-voltage electronics designed to function in extreme environmental conditions. The casing is highly resistant to dust, moisture and fluctuating temperatures, ensuring the devices can endure both scorching deserts and polar ice fields. Integrated environmental sensors can measure temperature, humidity and other key metrics, offering a holistic view of the ecosystem.
Yet it’s the AI component that truly sets this generation of camera traps apart. Instead of automatically transmitting every single photo, Instant Detect 2.0 employs an onboard machine learning model to filter out unimportant images—such as those of swaying branches or unthreatening animals. The system focuses on picking out specific “threat” signatures, for instance humans, vehicles or firearms. This reduces bandwidth usage and prolongs battery life, since sending satellite data is both power-hungry and costly.
Powering AI at the Edge
Developing an AI model capable of running on a device strapped to a tree (or frozen to an Antarctic outcrop) is no trivial task. High-speed servers found in data centres have ample processing power, but a rugged camera trap must rely on specialised low-power chips to conserve energy. The model used in Instant Detect 2.0 is trained on large, diverse image datasets containing various shapes, sizes and contexts for people, weapons and vehicles. Through a training process involving convolutional neural networks (CNNs) or hybrid architectures that borrow from transformer-based object detection, the system learns to differentiate everyday background wildlife from genuinely suspicious intrusions.
This training is typically performed on high-performance computing clusters so that the final neural network can be slimmed down and optimised for what’s known as “edge computing.” Reducing the model’s memory footprint is crucial: it needs to fit into the camera’s limited onboard hardware while still performing well against real-world noise—such as changes in light, weather conditions or partial occlusions (where an object is only partially visible).
Engineers and data scientists at ZSL and partner organisations continuously refine these algorithms. They explore techniques like quantisation, where the precision of calculations is carefully reduced to conserve processing power without severely affecting detection accuracy. The result is a flexible AI engine capable of distinguishing a ranger on patrol from a herd of zebra or a passing tourist jeep from a distant elephant bull.
Reaching Beyond Mobile Networks with Satellite Connectivity
Another significant technical leap is the integration of satellite modules. By interfacing with low-earth-orbit (LEO) or geostationary communication satellites, Instant Detect 2.0 can bypass local mobile networks completely. This is a game-changer for places like UNESCO World Heritage Sites located far from any telecommunications infrastructure, or marine protected areas in the middle of an ocean.
Once the system’s AI detects a threat, it compresses the relevant imagery and data, then transmits it via satellite back to a control centre. Alerts reach rangers or conservation managers within minutes. In practical terms, that might mean an anti-poaching unit receives a real-time notice on a smartphone or tablet showing a suspicious group entering the park. Rangers can then mobilise quickly, armed with precise location data rather than responding to a vague tip-off.
Why Being Open Source Matters
Unlike some proprietary camera trap solutions, Instant Detect 2.0 is being built to be open source. This ethos underpins both the hardware schematics and the AI software stacks. Conservation organisations across the globe can adapt the technology to their specific needs, whether it’s customising the detection model to recognise a local species or integrating new types of sensors, such as acoustic detectors for gunshot recognition.
Open-sourcing also fosters a collaborative environment where researchers and NGOs can share code contributions, exchange knowledge and improve the technology collectively. This benefits smaller conservation groups that may lack the budget to develop in-house AI expertise or navigate complicated licensing agreements. By lowering these barriers, ZSL and its partners hope to accelerate adoption worldwide, ensuring threatened habitats everywhere can leverage cutting-edge detection tools.
Putting Instant Detect 2.0 to the Test
Although still in advanced trials, Instant Detect 2.0 has already proven its adaptability. In Kenya, camera traps have been deployed for anti-poaching missions, alerting rangers the moment the system detects human figures or off-road vehicles in protected zones. In Antarctica, cameras are assisting in wildlife research, monitoring penguin populations and detecting any uninvited visitors who might disturb their nesting grounds.
These field tests demonstrate not only the robustness of the hardware but also the real-world performance of the onboard AI models. Data from these deployments feed back into the model’s ongoing development, helping engineers refine detection thresholds and reduce any false alerts triggered by unusual lighting or unexpected animal behaviour. As the dataset grows, so does the sophistication of the AI, laying the groundwork for truly global deployments.
Looking Ahead
Instant Detect 2.0 is a vivid example of how converging technologies—satellite communications, edge computing, machine learning and environmental sensing—can come together to tackle one of the most pressing environmental issues of our time. By granting rangers near-instant “eyes on the ground” without relying on traditional cellular infrastructure, the system stands to reshape how conservation is practiced in some of the planet’s most vulnerable areas.
With a planned rollout in the coming years, the Zoological Society of London and its collaborators are poised to make Instant Detect 2.0 available to a wider network of NGOs and wildlife authorities. If these technologies continue to evolve, we may soon see a world in which poachers struggle to hide from watchful camera eyes, remote habitats gain constant, unobtrusive monitoring, and critical conservation data is transmitted around the globe at the speed of light—even from the most remote corners of the Earth.
Further Reading
1. Zoological Society of London (ZSL) – Instant Detect Project Page
https://www.zsl.org/what-we-do/projects/instant-detect-2
Describes ZSL’s technology innovation in conservation, including the Instant Detect initiative and its evolution to version 2.0.
2. Instant Detect 2.0 Official Site
https://instantdetect.co.uk/
Provides an overview of system capabilities, development milestones, and trial updates directly from the project team.
3. Wildlabs – Instant Detect Case Studies
https://wildlabs.net/article/instant-detect-20-alpha-testing
Wildlabs is an online community for conservation technology. Here, you can find articles and case studies on Instant Detect and related field tests.
4. Nominet – Connectivity and IoT Collaborations
https://www.nominet.uk/zsl-adopts-nominets-open-source-iot-tools-to-help-wildlife-conservation/
Offers insights into Nominet’s work with ZSL on connectivity solutions for conservation, including their role in enabling satellite-based IoT systems.
5. Arribada Initiative – Open Source Conservation Tech
https://arribada.org/projectshttps://arribada.org/2020/02/17/progress-report-feburart-2020-thermal-imaging-for-human-wildlife-conflict/
ZSL collaborations on camera trapst.
6. WWF-UK – Supporting Partner
https://www.wwf.org.uk/project/conservationtechnology/camera-trap
Original Camera Traps
7. World Bank
https://thedocs.worldbank.org/en/doc/a5230ecc1d3b2784d8164137af1b9363-0320072018/original/SMaxwell-Conservation-Technology-03-21-18.pdf
Conservation presentation on Instant Detect(2018)