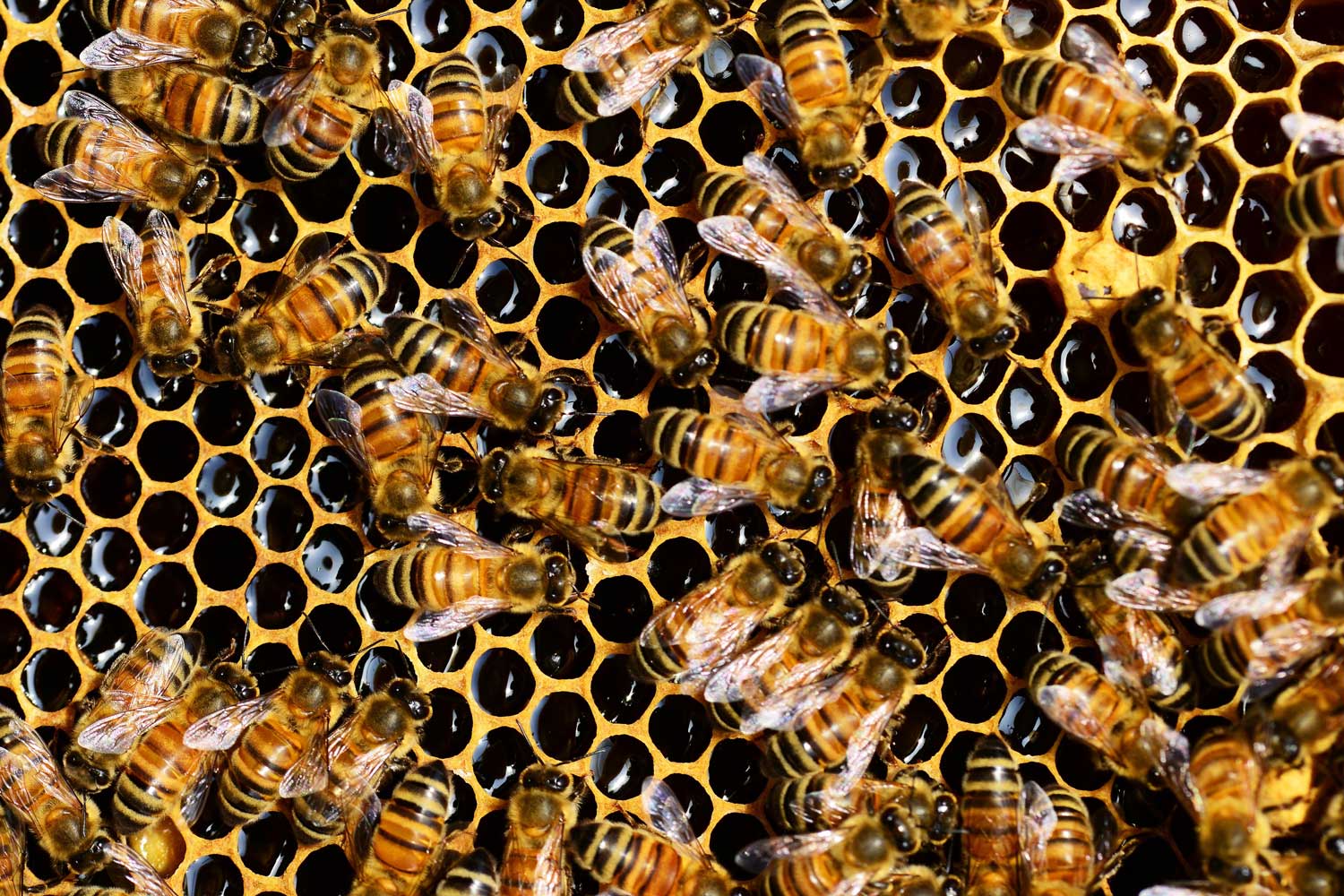
Understanding Neural Networks in AI
Introduction
Neural networks are an essential concept in artificial intelligence (AI) that often appear complex and challenging to grasp. However, understanding their fundamental principles can help demystify their role in AI. This articles breaks down the concept of neural networks and explains their importance.
What are Neural Networks?
A neural network is a computational model inspired by the human brain's structure and function. It consists of interconnected nodes or neurons organised into layers. These networks are designed to recognise patterns, learn from data, and make decisions or predictions, much like the human brain does.
Neural networks are particularly useful in AI because they can adapt and improve as they process more data. This ability to learn from experience allows AI systems to become more accurate and efficient over time.
Structure of a Neural Network
Neural networks are made up of three main layers:
- Input Layer: The input layer receives data and passes it on to the next layer. Each node in this layer represents an individual feature or variable from the input data.
- Hidden Layer(s): The hidden layer(s) are where the actual processing and learning occur. These layers contain nodes that perform mathematical operations on the data received from the input layer, extracting valuable information and patterns.
- Output Layer: The output layer is the final layer in the network, responsible for providing the result or prediction based on the information processed in the hidden layers.
The nodes in each layer are interconnected through weighted connections, which determine the strength and influence of one node over another. These weights are adjusted during the learning process to minimise errors and improve the network's performance.
Different Types of Neural Networks
There are several types of neural networks, each designed to tackle specific tasks or problems. Some of the most common types include:
- Feedforward Neural Networks (FNN): These are the simplest type of neural networks where information flows in one direction from the input layer to the output layer. FNNs are commonly used for tasks like regression, classification, and pattern recognition.
- Convolutional Neural Networks (CNN): CNNs are designed for processing grid-like data, such as images. They use convolutional layers to scan input data for local patterns, making them particularly effective in tasks like image recognition and computer vision.
- Recurrent Neural Networks (RNN): RNNs are specialised for processing sequences of data, making them ideal for tasks involving time series or natural language. They possess loops that allow information to persist, enabling them to maintain a "memory" of past inputs.
Creating and choosing a working Neural Network
While crafting a neural network from scratch is feasible, it's often more practical to select a pre-trained one from libraries like Hugging Face and adapt it to your needs. Read our in dept article on creating and choosing a Neural Network
Why are Neural Networks Important in AI?
Neural networks play a critical role in AI due to their remarkable ability to learn, adapt, and make predictions. Some reasons why they are essential include:
Pattern Recognition: Neural networks excel at identifying patterns and trends in complex, noisy data. This skill is valuable in numerous applications, such as image recognition, natural language processing, and fraud detection.
Adaptability: Neural networks can learn and adjust their performance as they encounter new data. This adaptability allows AI systems to improve and handle a wide range of scenarios.
Robustness: Neural networks can still provide accurate predictions even when faced with incomplete or noisy data, making them robust and reliable.
Scalability: Neural networks can handle large datasets and complex problems, making them suitable for a wide range of applications.
An Analogy
It can be hard to understand these concepts so we've written a separate article with an analogy to try and explain Neural Networks and Overfitting more clearly. The analogy is based on a team of high school teachers.
Conclusion
Neural networks are a vital component of AI, offering unparalleled pattern recognition, adaptability, robustness, and scalability. By understanding their basic structure and function, we can appreciate the potential of neural networks to drive innovation and revolutionise industries.