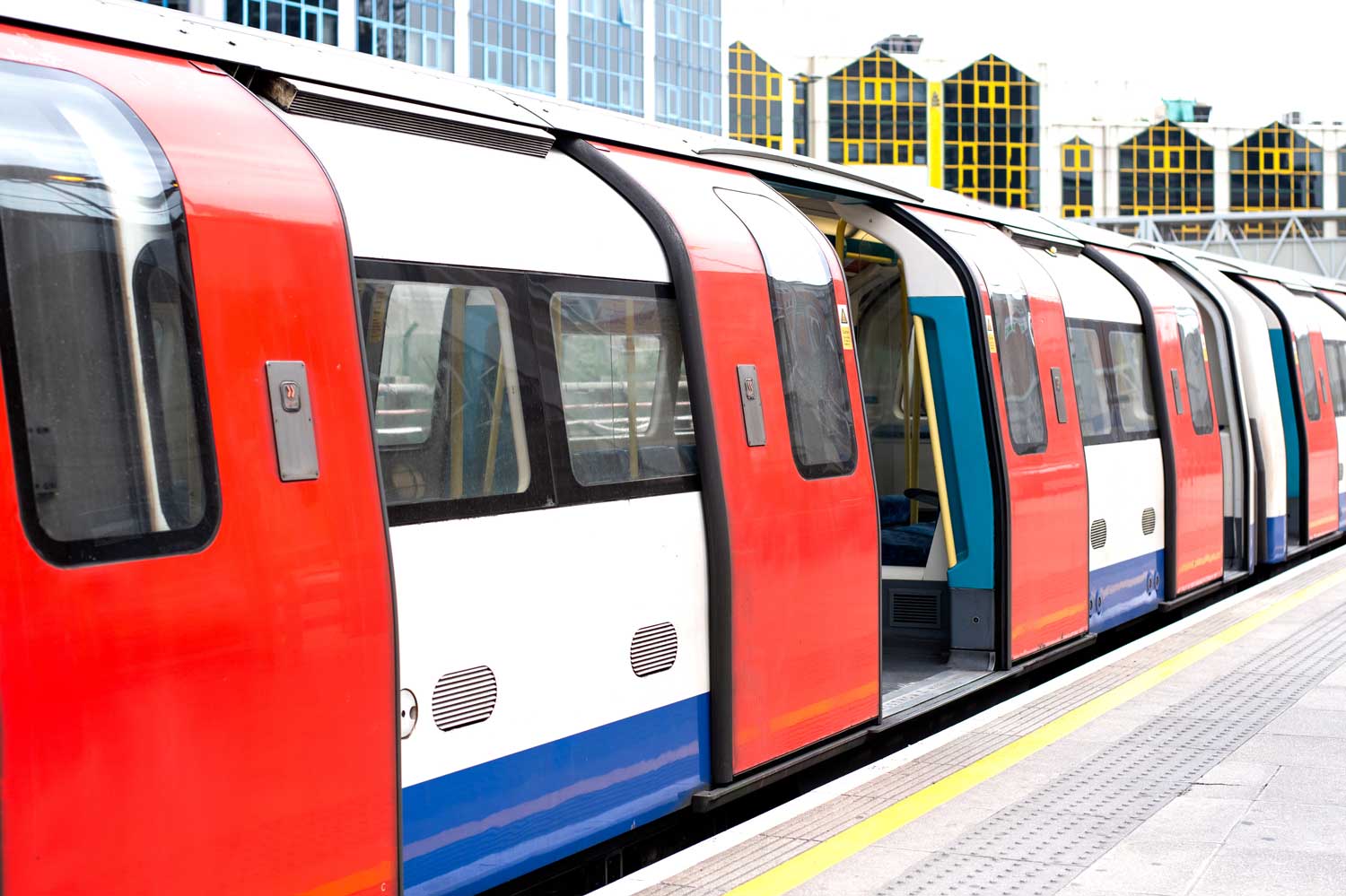
Using Artificial Intelligence to Improve Transport in London
Transport for London (TfL) is using artificial intelligence (AI) to improve the way people move around the city.
How Transport for London is Using AI to Enhance Public Transport
Transport for London (TfL) is responsible for the overall travel experience in London, managing and overseeing various modes of transport, including buses, the Underground, Overground, Docklands Light Railway (DLR), trams, and roads. While TfL facilitates these services, it aims to optimise them to reduce car traffic and promote walking, cycling, and public transport. To achieve this, TfL is looking at using AI to improve the way people move around the city. There are several key areas where TfL is leveraging AI: monitoring stations, preventing fare evasion and optimising road usage for cycling.
Leveraging AI with Existing Infrastructure
TfL has an extensive network of cameras already set up to monitor how people travel around London. These cameras provide a valuable resource for gathering data on movement patterns and behaviours. By integrating AI, TfL can use these cameras to:
- Analyse Movement Patterns: Understand how individuals move through different transport modes and locations.
- Enhance Safety: Detect unusual activities and potential hazards in real-time.
- Optimise Traffic Management: Monitor traffic flow and adjust signals dynamically to reduce congestion.
- Improve Operational Efficiency: Automate data collection and analysis to streamline decision-making processes.
Computer Vision
Computer vision is a specialised field within artificial intelligence (AI) that enables machines to interpret and understand visual information from the world. Central to this field are Convolutional Neural Networks (CNNs), a type of Neural Network, which are designed to mimic the human visual system . However, unlike humans, who might focus on specific areas of an image, CNNs analyse the entire image to detect patterns and features at various scales. This involves tasks such as:
- Image Classification: Identifying the subject of an image.
- Object Detection: Locating and recognising objects within an image.
- Image Segmentation: Dividing an image into segments to simplify analysis.
- Pattern Recognition: Identifying patterns and structures in visual data.
The CNN architecture effectively learns and runs the necessary algorithms to analyse and understand complex visual information, making computer vision a powerful tool for TfL's transport management.
Exploring Use Cases for AI in TfL
In order to determine how best to implement this technology across its network, TfL have identified different levels of use cases for AI, ranging from broader operational improvements to very specific tasks.
In this context, a use case is a specific scenario where technology is used to achieve a goal or solve a problem. It describes how users interact with the technology and how the technology responds to different inputs or events.
High-Level Use Cases:
Passenger Flow Management: Employing AI cameras to monitor and manage the flow of passengers through ticket barriers to reduce queuing times.
Safety Monitoring: Using AI to detect when passengers are too close to the platform edge or if someone appears to be in distress, enabling staff to intervene promptly.
Fare Evasion Detection: Using AI to monitor ticket barriers and identify passengers who attempt to pass through without paying.
Traffic Management: Analysing traffic patterns with AI to optimise traffic signal timings and reduce congestion on roads.
Cycle Route Planning: Using AI sensors to gather data on road usage by cyclists to plan and improve cycling infrastructure.
Specific Use Cases:
Spotting Discarded Items: Detecting discarded items on platforms to maintain cleanliness and safety.
Tracking Passenger Movements: Monitoring passenger movements to manage congestion and ensure efficient use of space.
Alerting Staff to Potential Dangers: Alerting staff if someone appears too close to the platform edge or shows signs of distress.
Detecting Raised Arms: Identifying raised arms as a potential sign of aggressive behaviour or distress, which can also serve as a discreet alarm mechanism for staff.
Recognising Wheelchair Users and Pushchairs: Spotting wheelchair users and pushchairs to provide support in stations without step-free access.
Identifying Knives and Guns: Training cameras to detect weapons with the help of British Transport Police to enhance security.
Monitoring Tube Stations
AI-powered monitoring at tube stations covers a wide range of use cases. Trials at Willesden Green and Blackhorse Road stations have shown how AI has the potential to make tube stations safer, more efficient, and more user-friendly.
At Willesden Green station on the Jubilee Line, TfL used AI-enabled cameras to create a 'smart station' by monitoring the entire CCTV network to identify various activities and potential issues. The AI monitoring was capable of detecting up to 77 different use cases, including spotting discarded items on platforms, tracking passenger movements, and alerting staff if someone appeared too close to the platform edge. This technology not only improved safety and cleanliness but also significantly enhanced operational efficiency by enabling staff to respond more quickly to issues.
The AI system at Willesden Green could also spot wheelchair users and pushchairs entering the station, allowing staff to provide support in a station without step-free access. The AI could also detect fare evasion, identify aggressive behaviour by recognising raised arms, and even spot knives and guns. Cameras were trained to identify these objects with the help of British Transport Police, who brought firearms to the station to train the AI.
During the trial at Willesden Green, TfL explored innovative ways to enhance safety using AI technology. One notable feature of the AI system was its ability to detect raised arms, a common gesture in aggressive situations. While this detection could occasionally produce false positives (such as people raising their arms for other reasons), it proved sufficiently accurate for identifying potential threats. TfL recognised an additional benefit of this capability: it could serve as a discreet alarm mechanism for staff. In scenarios where staff might find themselves in danger and unable to call for help using conventional methods, they could simply raise their arms. The AI system would recognise this gesture and alert the relevant authorities, ensuring that help would be dispatched promptly. This innovative use of AI highlights how technology can be leveraged to provide an extra layer of security, ensuring that staff have a reliable means of requesting assistance in emergencies.
Another successful AI trial was conducted at Blackhorse Road station on the Victoria Line. Here, an AI camera monitored ticket barriers and adjusted them based on passenger flow, reducing queues and increasing throughput by up to 30%. The trial demonstrated that if all barriers were automated, passenger throughput could be increased by up to 30%, and queue times reduced by up to 90%.
Fare Evasion Detection
Another critical use case for AI in TfL is catching fare evaders. Fare evasion is a significant issue for TfL, accounting for approximately 3.9% of all journeys and costing more than £130 million in lost revenue annually.
TfL and the British Transport Police (BTP) recently carried out a trial at Willesden Green station which demonstrated the effectiveness of AI in identifying passengers attempting to pass through barriers without paying. The trial used the station’s existing CCTV camera network to collect motion detection data. Advanced AI algorithms analysed this data to identify non standard behaviour and identify potential fare evaders in real time. The system then alerted staff so they could intervene.
One of the outcomes of the trial was the need to focus on ‘wide aisle’ tube gates. These are designed for passengers with luggage, pushchairs, or in wheelchairs and close slowly. The trial identified that these are often targeted people without valid tickets.
TfL and BTP have assured passengers that the AI system will be used solely for security purposes and that passenger privacy will be safeguarded. Siwan Hayward, TfL’s Director of Security, Policing, and Enforcement, emphasised that the technology is not being used as a ‘facial recognition tool’.
Traffic Management
TfL is implementing advanced AI technologies to enhance traffic management and reduce congestion across the city.
TfL has partnered with Siemens Mobility to develop and deploy the Real Time Optimiser (RTO) system, a cutting-edge upgrade to London's traffic control system. This new system aims to optimise traffic light timings, enabling smoother movement of people and goods on the road network while reducing delays and improving air quality.
The RTO system will integrate various new data sources and types of sensors to dynamically adjust traffic signal timings based on real-time conditions. This system can manage traffic more efficiently, especially during disruptions caused by incidents, planned works, or events. By analysing traffic patterns and using adaptive algorithms, the RTO system helps return the road network to normal operation quickly, thus minimising congestion and delays.
In addition to optimising traffic for vehicles, the system also considers cyclists and pedestrians. The RTO will introduce sensors that balance signal timings to accommodate high volumes of cyclists and pedestrians at busy locations, supporting TfL's Healthy Streets Approach, which aims to make sustainable travel more appealing and accessible.
Cycle route planning
Promoting cycling and improving infrastructure for cyclists is another important use case for AI in TfL. By using AI to gather insights on road usage before implementing new schemes and to analyse the effects afterward, TfL aims to achieve its goal of increasing walking, cycling, or public transport to 80% of all journeys in London within 20 years.
Historically, TfL depended on manual traffic counts to determine the number of cyclists on specific roads. These manual counts, conducted at limited locations across London’s road network, provided only a snapshot of road usage at particular times. To improve the detail and accuracy of cyclist data, TfL has trialled VivaCity sensors at two busy locations.
These AI-powered sensors operate 24/7 to detect and classify various types of road users, including cyclists, pedestrians, cars, and buses, with up to 97% accuracy. In addition to counting types of road users, they also record data such as time of day, speeds, and movement patterns, offering a comprehensive picture of how roads are used. This data is invaluable for planning new cycle routes and improving existing infrastructure, ultimately encouraging more people to choose cycling as a mode of transport.
Conclusion
By leveraging AI, TfL is poised to transform public transport, offering significant benefits for both passengers and the city of London.